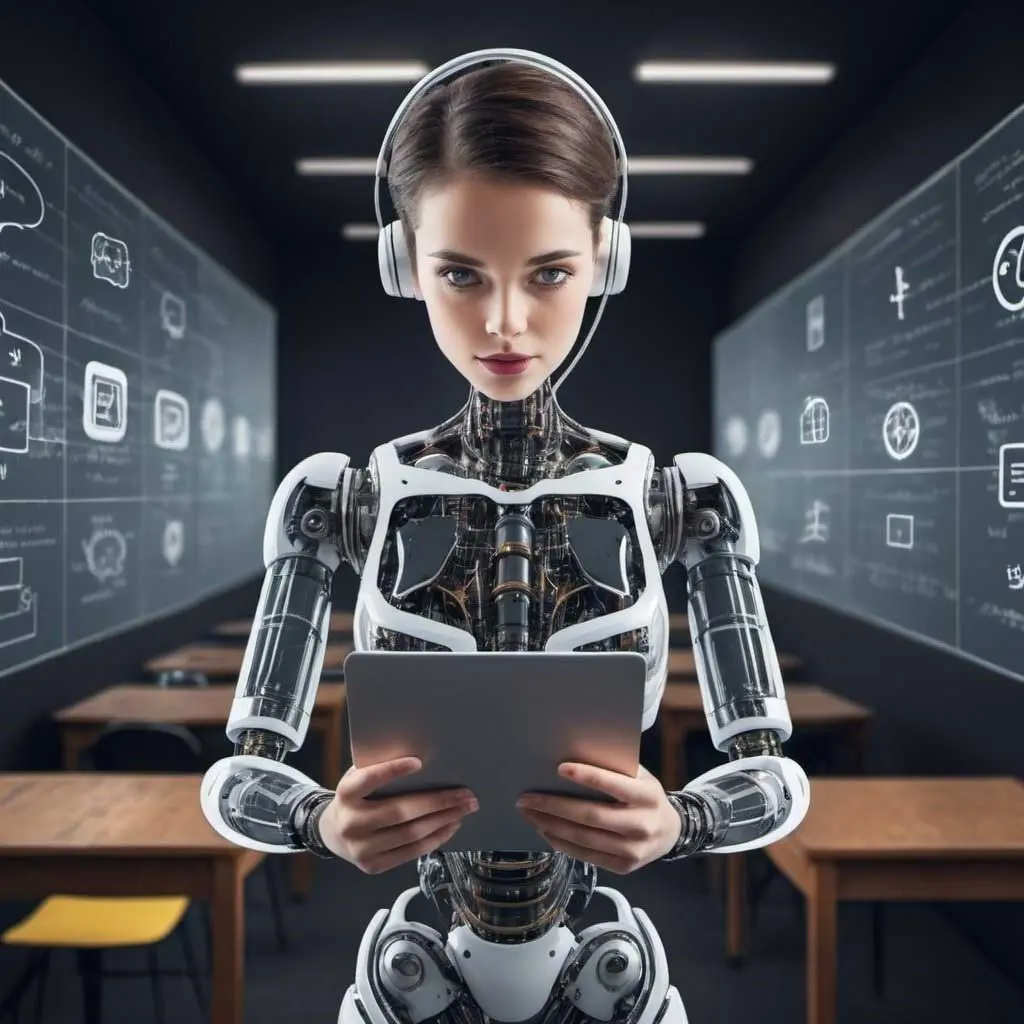
English in AI Technology
The role of English in AI technology is multifaceted and critical. English, being the predominant language in global communication, academia, and business, has naturally become the primary language for developing, training, and utilizing AI technologies. Below are several key aspects and applications of English in AI technology:
1. Natural Language Processing (NLP)
Natural Language Processing (NLP) is a core component of AI that deals with the interaction between computers and human language. The primary goals of NLP include reading, deciphering, understanding, and making sense of the human languages in a valuable way.
- Text Analysis: NLP techniques enable AI to analyze and extract meaning from text written in English, which is essential for applications like sentiment analysis, topic modeling, and summarization.
- Language Models: Large-scale language models, like GPT (Generative Pre-trained Transformer) and BERT (Bidirectional Encoder Representations from Transformers), are trained predominantly on English text data due to the abundance of resources and datasets available in English.
2. Machine Translation
Machine translation involves using AI to automatically translate text from one language to another. English often serves as a pivotal language in these processes:
- Intermediate Language: Many machine translation systems translate from a source language to English and then from English to the target language, due to the vast resources available for English language processing.
- High-Quality Datasets: The availability of high-quality parallel corpora in English and other languages enhances the accuracy and fluency of translations.
3. Voice Recognition and Speech Synthesis
Voice recognition systems, like virtual assistants (e.g., Amazon Alexa, Google Assistant, Siri), and speech synthesis technologies often rely heavily on English:
- Voice Command Systems: These systems are primarily trained to understand and respond to English commands, as English is widely used in technology and consumer products.
- Text-to-Speech (TTS): English TTS systems are well-developed and can produce natural-sounding speech, enabling applications in audiobooks, accessibility tools, and virtual agents.
4. Information Retrieval and Search Engines
Search engines and information retrieval systems leverage English due to the vast amount of digital content available in the language:
- Indexing and Search: English is often the default language for indexing web pages and documents, making it crucial for search algorithms and retrieval systems.
- Question Answering Systems: AI-powered question answering systems, such as those used by Google and IBM Watson, are trained extensively on English-language datasets to provide accurate and relevant responses.
5. Educational Tools and E-Learning
AI-powered educational tools and e-learning platforms often use English as the primary language for content delivery and interaction:
- Adaptive Learning: Systems that tailor educational content to individual learning styles and progress frequently use English-language content due to its broad reach and resource availability.
- Language Learning: English is a common focus for AI-driven language learning apps, which use speech recognition, grammar checking, and interactive exercises to teach English.
6. Sentiment Analysis and Opinion Mining
Sentiment analysis involves detecting the sentiment expressed in text, which is commonly applied to English text data due to the volume of user-generated content in English:
- Social Media Monitoring: AI systems analyze English posts, comments, and reviews to gauge public sentiment and trends.
- Customer Feedback: Businesses use sentiment analysis to assess English-language customer reviews and feedback to improve products and services.
7. Ethical Considerations and Bias Mitigation
Given the dominance of English in AI development, there are ethical considerations and challenges related to bias and representation:
- Language Bias: AI systems trained predominantly on English data may exhibit biases, overlooking nuances in other languages and cultures.
- Inclusion and Diversity: Ensuring that AI systems are inclusive and fair requires addressing these biases and expanding training datasets to include diverse languages and dialects.
Conclusion
English plays a crucial role in the development and application of AI technologies. From NLP and machine translation to voice recognition and sentiment analysis, the prominence of English in digital content and communication makes it a foundational element in AI research and deployment. However, it’s essential to acknowledge and address the biases that can arise from an over-reliance on English data to create more inclusive and representative AI systems.